AI and football make a beautiful game
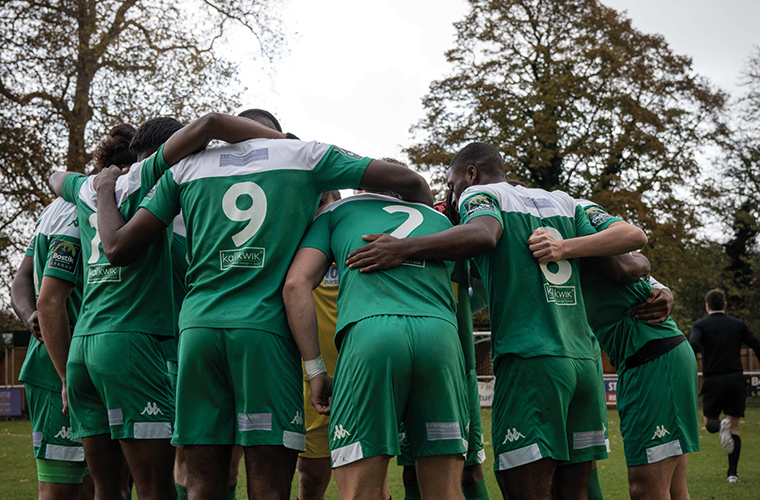
‘Hey Watson, is my football team going to win this Saturday?’ The IBM Player Spotlight can’t tell you this – yet – but it can help sports teams improve, while enhancing the viewer experience through added content. We find out how it all began…
On the final weekend of the Fifa Women’s World Cup France 2019, broadcasters across the globe enjoyed record audiences as viewers flocked to watch the most important match in women’s football.
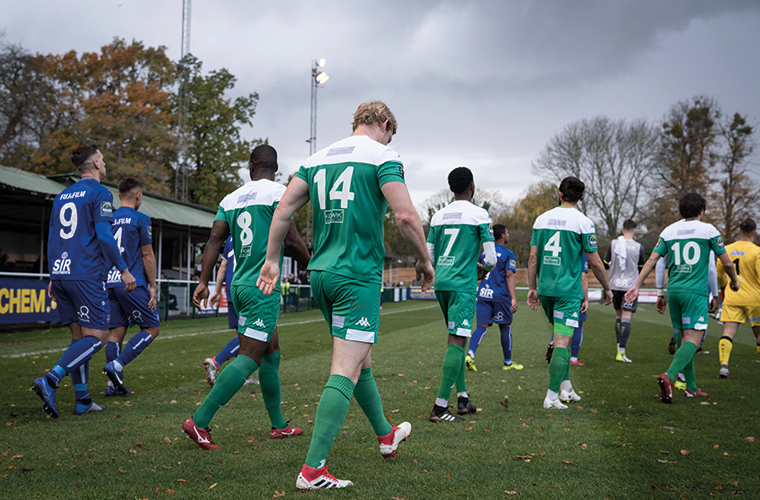
IBM Player Spotlight was first used by football club, Leatherhead FC, to examine match reports and social media feeds
In the US, the defending champions’ victory over the Netherlands achieved a combined audience of 15.277 million viewers – the highest audience of the weekend on US television.
These millions of viewers not only enjoyed watching their country being crowned World Cup champions, but their viewing experience was enhanced with access to player data, statistical analysis and key insights into individual player’s performances and the teams.
This was made possible thanks to IBM Player Spotlight, a new product from IBM, which uses the company’s Watson artificial intelligence technology, and was utilised by Fox Sports during the Women’s World Cup to access statistical analyses using an easy-to-use conversational interface.
We had to give it a few examples of ‘corner’ being used in different ways… but it evolves over time with the more examples and the more training data you give
The solution uses an instance of IBM Watson Assistant that has been trained to understand football-specific terms including team names, player names, locations on the pitch and events in the game. With Watson, Fox Sports’ commentators could pull trends and statistics comparing individual and team performance. The Player Spotlight system ingests detailed tournament data from Opta, a data provider that collects and analyses every pass, goal and penalty kick. The commentators then interact directly with the data by asking natural language queries of Watson, which then presents highlights, data and analytics.
“Every human can use natural language,” explains Joe Pavitt, master inventor at IBM. Using the AI-based system, “commentators would simply ask questions about England’s or the US’ performance using a conversational interface. They would then get video and stats coming back that they would use in their pregame show.”
Examples of questions that Watson can understand are, ‘show all the shots for the US in the second half’, ‘show the players that are involved the most for England’, and ‘what is the pass network for France shots?’
Watson replies with analysis and stats, allowing Fox Sports to uncover insights into the match that would have previously taken hours to find.
Across the pond
Like an actual player, Watson has made its way up through the ranks, beginning its football career with Leatherhead FC, a 7th tier English football club.
Leatherhead FC looked to Watson to examine match reports and social media feeds in order to gather information and analyse the opposition to provide a comprehensive view of their opponents’ recent games and how they might fare against them in their next match.
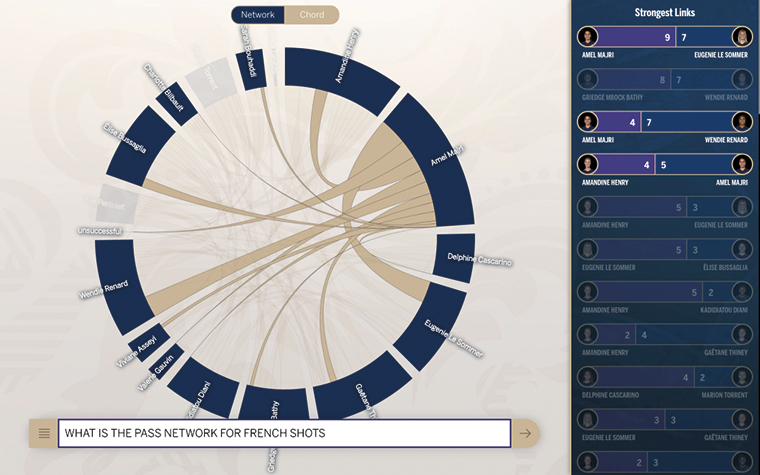
During the Women’s World Cup, Fox Sports provided viewers with players’ stats, match trends and analysis
“The Leatherhead management didn’t care what AI was,” says Pavitt, “they just wanted access to data. The players, coaches and management were using this, so it was important to understand how people wanted to interact with Watson. The main task was teaching football terminology to Watson so users could ask questions along the lines of, ‘how do the Leatherheads make shots?’”
AI differs from traditional applications in that it gets better the more it is used. “You don’t programme Watson, you teach it,” says Pavitt. “The more you train it, the more it can answer.”
Towards the end of the season, Watson had learned enough to provide customised video presentations around individual players
IBM worked with the team’s management to develop a system to assist their match analysis and opposition scouting. The platform gave the coaches and players easy access to video footage, Opta data, insights and analytics.
The training began with the IBM team asking the Leatherhead managers to write down five different ways they would ask somebody for shot data and then train Watson with those terms. This was then tallied up with the match metadata from Opta, which was time-stamped with the video footage.
“Watson learns and understands over time,” explains Pavitt. “We had to give it a few examples of ‘corner’ being used in different ways. For example, ‘player A takes the ball into the corner’ – refers to an area of the pitch in open play, while, ‘player B puts a corner into the box’, refers to a football set piece – a player kicking from a specific place. Once you give it those different examples, it will start to learn. The natural language agent, IBM Watson Assistant, was trained purely on text and examples along the lines of ‘show me every time player A did X’, and the different ways that can be phrased. It then tries to match an unknown phrase with what it does know – see where it fits. It evolves over time with the more examples and the more training data you give.”
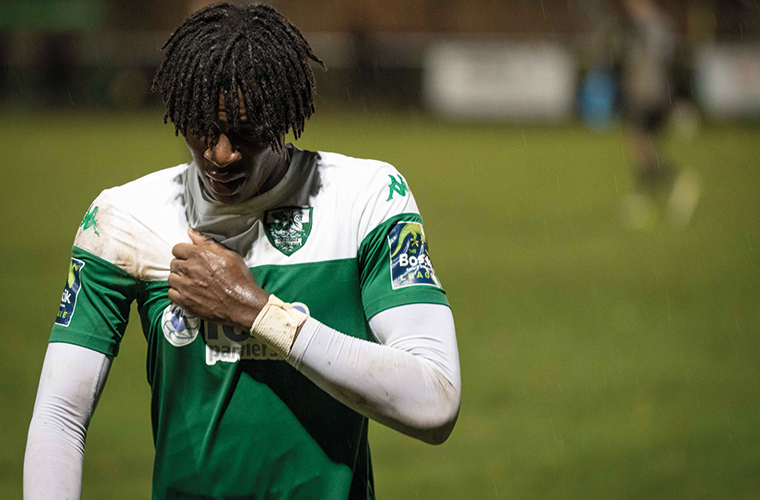
Leatherhead FC players began using IBM Player Spotlight themselves to improve their performances
Watson is trained with historical footage from games, as well as custom statistics that were generated in-house from IBM’s own understanding of the game. “As such, we are able to group together events from the Opta dataset to form a ‘play’. These plays are not only limited to a particular event, for example a goal, but will show the context of the build-up to that goal, how the play started and resulted with the ball going into the net,” says Pavitt. “Having these plays in place, we are then able to apply another model to categorise the style for a particular play. For example, is this showing us wide play? Is this ‘route one’ play? Now that we have this high-level classification for a play, as well as all the events that make up that play, we have more context to work with. This enables us to present the most useful information to the user in each scenario, be it as video clips of a particular play or breakdowns of areas of play on the pitch; areas where a team are more vulnerable.
“This holistic view of all the data in one place provides huge opportunities for analysis and insight by the users. With it all in place, a manager could say to Watson, ‘show me all the shots from Saturday’, and Watson would create a playlist of the shots from that match. Towards the end of the season, Watson had learned enough to provide customised video presentations around individual players.”
The system was designed to be used by the manager and assistant manager, however “once the players found out it existed, and how easy it was to use, they began to ask for access themselves”. Pavitt adds: “As the season went on, different players had different ways of asking for things – so it evolved. The assistant manager said to us, ‘you could tell which players were using it and which players were not using it’.
“If a player wanted to use the tool, that implied the player wanted to get better. That helped with the selection of the team.”
IBM Player Spotlight has opened up a new avenue for not only players and teams to explore complex performance data, thus hopefully improving their own performances, but for sports broadcasters to receive answers to their questions in seconds and deliver added content to their audiences almost instantaneously.
Now if only Watson could help our football team win the Premier League
this season…
This article originally appeared in the September 2019 issue of FEED magazine