Masterclass: Maximising Machine Learning
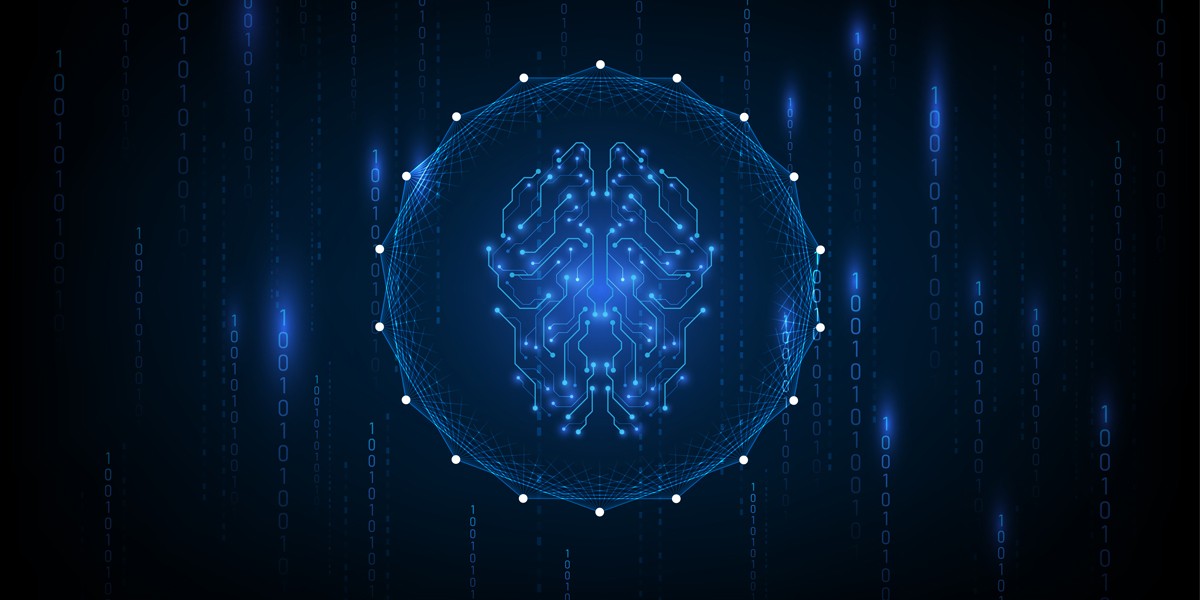
Posted on Jul 20, 2024 by FEED Staff
Machine learning΄s presence is felt in every corner of the broadcast landscape. This issue΄s panel explores the deeper meaning behind its impact
The experts
- Michael Cioni Founder and CEO, Strada
- Tim Jung Founder and CEO, XL8
- Harry Bloxham Head data scientist, Scan Computers
- Brian Kenworthy Vice president of media operations, TMT Insights
Can you share the difference between ML and AI?
MICHAEL CIONI: The debate of the difference between machine learning (ML) and artificial intelligence (AI) only exists in the academic space. A consumer should think of them as the same thing and not worry about the disparities.
Technically, ML is the technique of taking existing data, analysing it and generating an output a computer can use to recognise patterns. A simple example is auto-complete; the computer analyses a bunch of text and determines how common it is for a word to come after another.
A simple ML algorithm might just use the previous word to detect the next word, but when you are writing a sentence, it can typically struggle to accurately predict the actual word you intend to use. A more complex algorithm can look at entire sentences, paragraphs or pages and use that information to more accurately determine the word you intend to use.
Simply put, if the algorithm didn’t have any examples of the word you intend to use coming after your previous word, it could never suggest your intended word.
That is the key difference between ML and AI. AI is intelligent and capable of understanding. It is a metaphysical concept, as it doesn’t need a previous example to know what comes next; it can invent and create.
The lines have become progressively blurred with further developments of ML. As the complexity of the algorithm grows, it begins to combine seemingly unconnected elements, demonstrating the perception of intelligence.
BRIAN KENWORTHY: Although ML and AI are closely related concepts, they are still distinct in their own way. AI is a broader category which uses machines to automate processes or enable machines with intelligent behaviour. ML, on the other hand, is a specialised branch within AI which focuses on systems to learn from data without explicit programming. While AI aims at automating tasks and decision-making, ML specifically focuses on pattern recognition and predictive modelling through data analysis.
HARRY BLOXHAM: AI is the umbrella term for the designing of systems to mimic human intelligence. It is broken down into subsets including ML and deep learning. With ML, the target is to create a simulation of human learning which allows an application to adapt to uncertain or unexpected conditions. Think of unstructured data, like applying metadata to clips or facial recognition.
To perform this task, ML relies on algorithms to analyse huge datasets and perform predictive analytics faster than any human can. It uses various techniques including statistical analysis, finding analogies in data, using logic and identifying symbols. In contrast, deep learning processes data using computing units called neurons, arranged into ordered sections known as layers. This technique – at the foundation of deep learning – is called a neural network and is intended to mimic how the human brain learns. It is this deep-learning technology that is commonly (perhaps incorrectly) called AI today.
TIM JUNG: ML comprises algorithms and methodologies which enable software programs to learn from data – either labelled or unlabelled – without requiring explicit programming for specific conditions by human engineers. In contrast, AI is a broader term encompassing systems mimicking human intelligence, including ML and explicitly programmed software. Deep learning – a subset of ML – structures its computational models similarly to how neurons are interconnected in the human brain.
The precise distinctions among these terms have become quite blurred recently, particularly to the general public. This is because the recent advancements in deep learning have led to its predominance within the field of ML, and consequently, some people started using the terms interchangeably.
Nowadays, the term AI seems to be used more in the marketing space to attract general attention, while ML is used more in the technology space to specifically describe the type of ML algorithms being used.
How has ML evolved the media-tech landscape in the past ten years?
HARRY BLOXHAM: ML algorithms and the productivity tools they spawned have led to automation of many intricate tasks which were once time-consuming, and these have been in applications for a while. Adobe Sensei introduced tools like content intelligence and face-aware editing, while the Neural Engine was added to DaVinci Resolve 16 with upscaling and auto colour.
These meant that even independent artists and creatives could benefit from simpler and slicker workflows – the iterative process is still required but the timescales and complexity are both reduced. Over the years, as these tools have evolved for almost every stage of the creative process, efficiency has been impacted significantly in completing projects faster while using less resources at the same time.
Jumping to the last couple of years, this has only accelerated as we’ve seen generative AI tools integrated into applications. For example, the ability to input prompts with tools like Adobe Firefly allows users to automatically add frames and remove objects.
Outside of this integration, platforms like Nvidia Omniverse have brought the ML backbones of many individual tools into a collaborative real-time environment, providing a significant boost to efficiency.
TIM JUNG: While the media industry seems closely aligned with technology, its adoption of tech has ironically been slower compared to sectors like e-commerce, logistics or home appliances. This cautious approach resembles industries where accuracy and safety are crucial, such as healthcare and automotive. Traditionally conservative, the media industry has gradually adopted technologies like cloud-based storage and software systems.
This trend extends to ML as well. I categorise ML systems by their level of autonomy: firstly, ML as an alarm, where ML alerts humans who then take action; secondly, ML as a tool, where humans utilise and refine ML-generated suggestions; and lastly, ML as an agent, where ML independently performs tasks.
Until 2018, ML in the media was primarily used as an alarm, for functions including broadcast monitoring and detecting censorship violations. However, the landscape is rapidly changing; we now see ML utilised as a tool for creating computer graphics, transcriptions and subtitling, where human experts refine the drafts produced. Some ML algorithms have greatly enhanced user experience on media platforms through ranking, recommendations and personalisation. More recently, ML has moved into real-time applications where post-editing by humans isn’t feasible.
What are some of the most exciting innovations you΄ve seen in the ML space?
BRIAN KENWORTHY: One of the most thrilling innovations in the ML space is personalisation. It revolutionises user experiences by tailoring content recommendations and advertisements based on individual preferences and behaviour patterns. Whether it’s suggesting movies aligned with viewing history or presenting targeted ads matching personal interests, personalised content delivery enhances engagement and satisfaction.
Plus, ML-powered content creation tools introduce remarkable efficiency gains. Auto rotoscoping, editing and commercial break placement streamline production workflows, while flagging censorship scenes ensures compliance with regulations. Moreover, ML-driven restoration techniques breathe new life into older material, like The Wizard of Oz from 1939. By removing noise from audio and cleaning up imperfections, restoration ML enhances the visual and auditory quality, providing audiences with a pristine viewing experience in the modern era.
MICHAEL CIONI: The most exciting innovation in the ML space has to be around globalisation of content. Connecting with people across the world is something the internet has fostered. We can exchange information and interact with each other at the speed of light – but still have a huge problem: we don’t all speak the same language.
ML now offers the ability to both translate text as well as media content such as audio and video. People across the world have dramatically different experiences, inspirations and cultures. This type of ML technology enables us to enjoy stories, viewing the content just like it was made in our own language – but in reality, the content was made in a language we may not know a word of.
What΄s your favourite ML application, and why?
BRIAN KENWORTHY: I particularly like speech recognition, generation and auto-translation. These tools play a pivotal role in democratising content accessibility, breaking down barriers to information and entertainment for global audiences. Through automatically transcribing, translating and generating content, ML empowers individuals to engage effortlessly with a wide array of media content – regardless of linguistic or cultural differences.
This democratisation not only enhances inclusivity, but also fosters cultural exchange and understanding on a global scale. By reducing reliance on manual translation processes, these tools alleviate the workload of human translators, allowing them to concentrate on more intricate and culturally nuanced projects. In essence, the democratising effect of ML in broadcasting transcends linguistic boundaries, promoting a more interconnected and culturally enriched media landscape.
TIM JUNG: When this question is directed at someone committed to bridging language barriers, the response is predictable. In 2020, we launched the world’s first live subtitling solution. From the outset, this feature garnered enthusiasm, especially during an online fan meeting between a K-pop idol and their international fanbase. Previously, these fans had watched the shows without fully understanding the discussions. Our real-time translation transformed their experience, allowing them to engage deeply with discussions.
The tech has continued evolving massively. We have achieved major advancements in real-time translation. Generative AI has enabled us to automatically incorporate glossaries specific to the topic at hand.
Michael Cioni: My favourite application of ML in broadcast is in sports. For years, we have heard this joke that watching sports at home is better than the stadium. With the advancement in real-time analytics, this becomes even more true. You can know how fast someone ran, how high they jumped or the probability that the play that happened was supposed to happen. It’s extremely engaging, interesting and connects the viewer to the game in an entirely new way.
Does ML present any challenges to the media-tech space?
MICHAEL CIONI: Any new technology will present challenges in any sector; ML will not be immune to this. The new opportunities will not always outweigh the costs until the tech develops to solve the problems it created.
One of the biggest challenges will be in economics. We have seen large projects make billions of dollars but cost hundreds of millions to make. If you take it down a scale, it has historically been difficult to spend thousands of dollars and make millions. Essentially, the largest monetary gain was in the big projects and the studios were the only ones who could output the millions to get the big payoff.
ML offers a promise for a creator to spend thousands and potentially make millions. While millions is not billions, the initial investment isn’t required and the profit margin can be much higher. This will cause many challenges to the media-tech space, as it will have to adapt to the changing market conditions. Teams will work differently, be of different sizes, have different requirements and demand innovation from the technology they use.
HARRY BLOXHAM: While there is often fear and trepidation surrounding the adoption of AI and ML in all industries, not just media – generally around jobs being replaced – the better way to view it is as the introduction of a whole new suite of tools to help make your tasks simpler and more efficient. There is one major challenge to the adoption of these tools in the media space: the models are often based on open-source data. The issue comes with the data; to amass the quantity required, it is often simply collected from the internet and will include copyrighted data, which leads to ethical and legal issues in its commercial use.
What other opportunities lie ahead for ML in broadcast?
TIM JUNG: As more processing power is added and more ML models are optimised for real-time use cases, we can expect broader adoption of ML algorithms in the broadcasting industry. These applications can range from validation and analysis to production and post-production processes.
Real-time broadcasting monitoring offers significant opportunities for censorship compliance, copyright management and delivering personalised ads. Expanding viewership through real-time transcription and translation – along with the integration of sign languages – will greatly enhance accessibility. In the near future, content might be entirely or partially created by ML models in real time, such as generating video streams from radio broadcasts.
HARRY BLOXHAM: Although we’ve mentioned the automation, efficiency and collaboration delivered by ML-powered applications and tools, it may be the combination of these that holds the greatest opportunity. Taking virtual production as an example (an area which has grown massively in recent times), there used to be significant demands on time and resource to work together in various locations. It’s been well covered that virtual production brings the location to the studio in almost real time, offering huge benefits.
Yet behind the scenes – as post-production is being brought earlier in the pipeline to create the environments on a virtual production set – the advancements in ML are only making it easier for flexible contributions. The ability to create and work together in digital spaces, accelerated and enhanced by AI, may well revolutionise media production as we know it today.
BRIAN KENWORTHY: The future for ML in broadcast holds an explosion of content creation and innovation driven by a more democratised creative community.
Automated editing, captioning and metadata generation will streamline workflows, allowing creators to focus on the creative and produce amazing, high-quality stories for broadcast. This streamlined process enables higher-quantity creation of high-quality stories for broadcast, expanding opportunities for creators across diverse backgrounds.
Additionally, ML technologies will optimise audience reach by tailoring content to individual preferences, fostering deeper engagement and loyalty across the board.
MICHAEL CIONI: There will be extensive usage of ML in the future. Technology companies will need to focus on the latency and stability demands of broadcast – and that will take some time. The future of the broadcast landscape will live or die by personalisation.